Research degrees
PhD opportunities
Visit phd.leeds.ac.uk to search our project ideas, funding opportunities, research areas and PhD supervisors
Search phd.leeds.ac.uk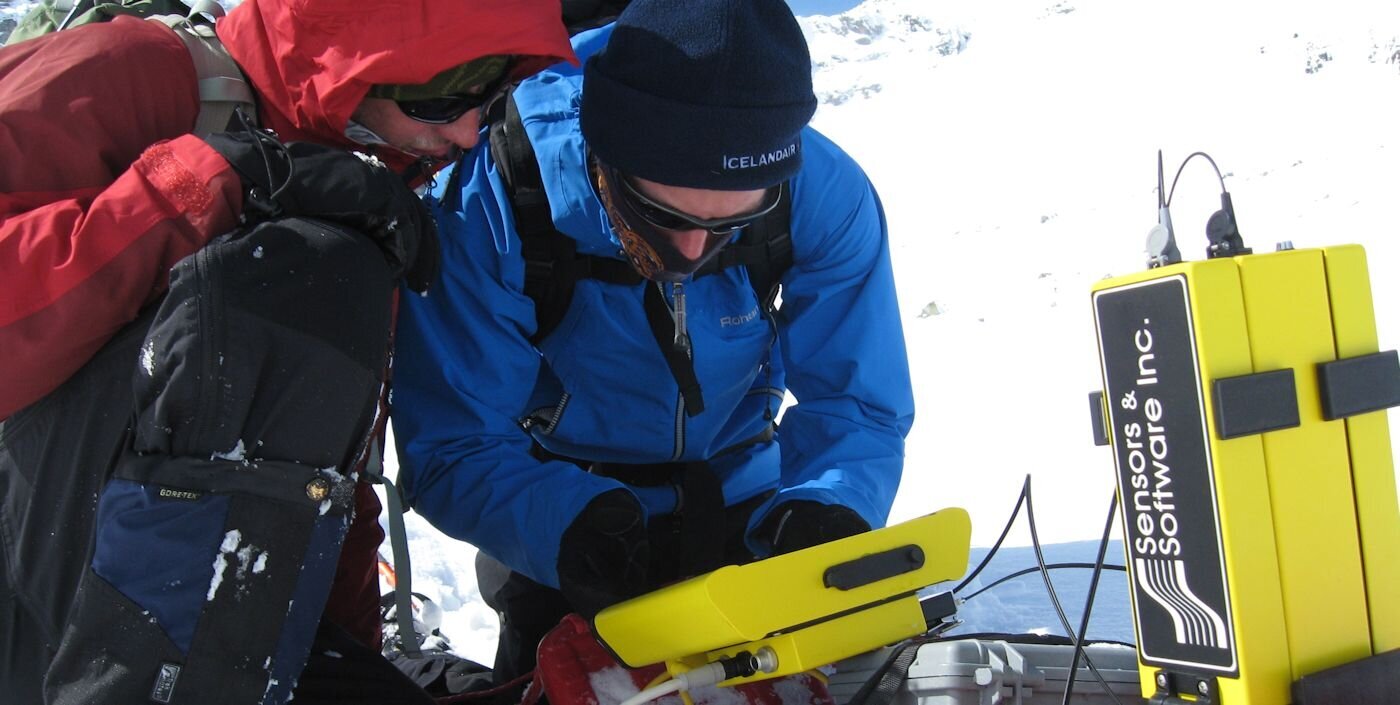
Research degrees
Whether you’re looking to begin an academic career or want to develop your skills and expertise for a career in industry, studying for a PhD will be a journey that will stimulate your passion for your subject and push you to reach your potential.
We offer different types of degrees including full and part-time PhD degrees, split-site PhD, MPhil and a Masters by Research.
The structure of a typical PhD, usually takes between 3 to 3.5 years full-time or 5 years part-time, during which you’ll be generating new knowledge and considering that new information in relation to existing information. You’ll need to be dedicated and passionate about your area of study. But it’ll be well worth it. Being a doctoral student is challenging, but incredibly rewarding.
Postgraduate research in the School of Geography
Why do a research degree at Leeds?
Study in an active research environment: The School of Geography is globally renowned for the quality of its research, and the funding it attracts, meaning we’re able to invest in world-class facilities and academic staff who are actively engaged in cutting-edge research. The results of the 2021 Research Excellence Framework (REF2021) show that 93% of the University of Leeds’s Geography research submitted was rated as either ‘world-leading’ or ‘internationally excellent’.
A strong networks of support: The Leeds Doctoral College connects our whole researcher community and put you in touch with the services, guidance and opportunities you need. From day one, you’ll benefit from a strong support network in the School of Geography to guide you through your research degree.
Professional skills development: We think of the whole picture at Leeds. That’s why we offer a range of workshops and courses that'll enhance your skillset further and transfer into your professional career.
Close industry links: You’ll have the opportunity to attend and present at conferences and research events where you’ll meet and network with colleagues from industry and academia. You’ll benefit from our close working relationships with a large number of key companies and academic institutions in the UK and overseas.
Global community: You’ll join a friendly, supportive and diverse community of students and researchers who come from all over the world and have access to wellbeing and support services throughout your degree.
Applying for a research degree
How to write a research proposal
Here's how to produce a document that outlines your proposed research topic and programme of research
More on How to write a research proposalHow to apply
Whether you're applying to a project or proposing your own, follow this step-by-step guide to completing your application.
More on How to applyFees
Find out our research degree fees. If you are a former student of the University of Leeds you may be eligible for a 10% alumni tuition fee bursary.
More on FeesEntry requirements
Find out our PhD and MA/MSc Masters by research entry requirements in the School of Geography.
More on Entry requirementsDoctoral Training Programmes
The School of Geography contributes to a number of structured PhD training programmes which focus on interdisciplinary research. Funding is currently available through the White Rose ESRC Doctoral Training Centre and the Panorama NERC Doctoral Training Partnership offer a number of funded projects. The Centre for Satellite Data in Environmental Science CDT (SENSE) also offers funded projects for PhD researchers to harness satellite data to tackle global environmental challenges. The new Extinction Studies DTP, funded by the Leverhulme Trust, fundes a numbers of doctoral researchers per year to explore responses to the global extinction crisis.
My PhD experience
Fran Pontin
Working in a data partnership with Active Inspiration, who develop mobile app technology to help people get more active, has allowed me to develop my understanding of the data generation process.
More on Fran PontinKeiran Suchak
Many of the PhD projects run by the CDT for Data Analytics and Society are run in conjunction with external partners; in my case, my project is run with Leeds City Council.
More on Keiran SuchakDr Alice Noble
Completing my PhD at the University of Leeds with Natural England as a partner was a fantastic experience.
More on Dr Alice Noble